
We are data: we are one, we are many
What happens if an algorithm arbitrarily decides whether a loan is approved, or an individual is labeled as a “risk” for society? It is a fair decision? After all, the algorithm is exercising its function of control through logic (Fuller, 2008, p. 15). It is taking objective decisions based on the data it gathered on categories of people like the one under scrutiny at any given moment. One could argue that the data that is gathered is flawed or, more simply, that human beings do not always fit within categorical boxes. Then, perhaps, an issue lies within the way data are gathered, and what the algorithms is instructed to do by its human coders and owners. Humans’ actions are constantly monitored on online spaces, and some are fine with it as they “have nothing to hide”. However, this apparent inability to understand the consequences of constant surveillance online can be harmful not only to those who make their extraction of data extremely easy, but especially to those who share similar characteristics with them, who will be labeled the same way, put in the same “box”, treated the same preemptive way.
What does “we are data” mean?
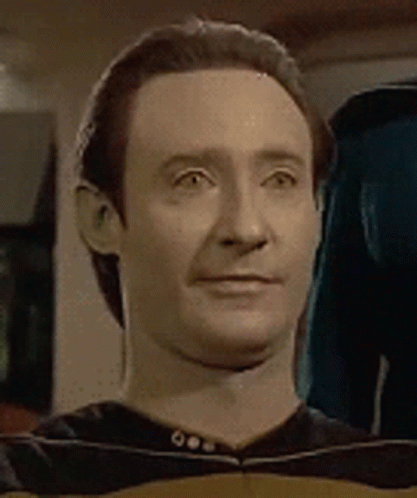
No, it doesn’t mean that we are becoming androids like Lieutenant Commander Data from Star Trek: The Next Generation. We are, however, losing our agency and our subjectivity, as the virtual does not treat us as unique people, but we become different persons according to the characteristics (that are part of us) that algorithms are looking for at a particular moment (Cheney-Lippold, 2017, p. 10).
Issues with being treated as data
Algorithms are flawed. Even the most effective one has mistakes in its code every 10000 or so lines (Christl & Spiekermann, 2016, p. 126). These mistakes allow unconscious human bias to emerge and cause discrimination and exclusion when algorithms are in action “in the wild”.
A critical issue in being considered data is the big data divide, an asymmetric relationship between users and corporations: we don’t have access to big data and, even if we did, we do not have the skills or means to interpret it (Andrejevic, 2014, p. 1674). Adding to this, we mainly only have a vague idea of the inputs that make society work and progress, but we do not know how there inputs interact among them to obtain certain results (Pasquale, 2015, 3). Thus, some people are familiar with what algorithms are, but most are unaware of the implication these packets of software can have daily on people. Scholar Tressie McMillan Cottom better explains the impact of being racially profiled data with the concept of predatory inclusion: the logic of “including marginalized consumer-citizen into ostensibly democratizing mobility schemes on extractive terms.” (McMillan Cottom, 2020, p. 443) With this, she means that in order to keep the market profitable, marginalized individuals are invited to succeed in life, but at a high cost: for example, being able to access higher education with a very high interest loan.
Lastly, a recent development about transactions between military government and seemingly innocuous apps unveiled the extraction of location data of millions of users; in one particular case, they were all Muslim, from a Muslim prayer app. This clearly denotes a breach of privacy that goes well beyond the harm of a single person. Indeed, with that data it will be possible to further racially profile any future person that declares to be Muslim online, as the algorithm will pick up that trait and disregard all others that might still be relevant. In so doing, the individual will lose their uniqueness, and will be categorized only on the basis of their faith. We are data, but some is more lucrative than other.
________
Bibliography
Andrejevic, M. (2014). The big data divide. International Journal of Communication, 8, 1673-1689.
Cheney-Lippold, J. (2017). We are data. Algorithms and the making of our digital selves. New York University Press.
Christl, W. & Spiekermann, S. (2016). Networks of control. A report on corporate surveillance, digital tracking, big data & privacy. Facultas.
Cox, J. (2020, November 16). How the U.S. military buys location data from ordinary apps. Vice. https://www.vice.com/en/article/jgqm5x/us-military-location-data-xmode-locate-x.
Goffey, A. (2008). Algorithm. In M. Fuller (Ed), Software Studies: A Lexicon (pp. 15-20). MIT Press.
McMillan Cottom, T. (2020). Where platform capitalism and racial capitalism meet: The sociology of race and racism in the digital society. Sociology of Race and Ethnicity, 6(4), 441-449. DOI: 10.1177/2332649220949473.
Pasquale, F. (2015). The black box society: The secret algorithms that control money and information. Harvard University Press.
[Untitled gif of Lieutenant Commander Data]. Tenor. https://tenor.com/view/star-trek-data-crazy-tng-next-generation-gif-18122119.